The goal is now in sight – if not yet in reach: a fully-automated operational production environment.
The rise of DevOps shows the progress we’ve made in automating the provisioning and configuration of ops, as well as application deployment. Management of the ops environment isn’t far behind.
IT Operations Management (ITOM), and in particular Application Performance Management (APM) are now well on their way to realizing this hands-off vision. But we’re not there yet. In today’s complex enterprise production environments, we still need people – but as the environments and applications become more difficult to manage, we must give our ops personnel smarter, more powerful tools.
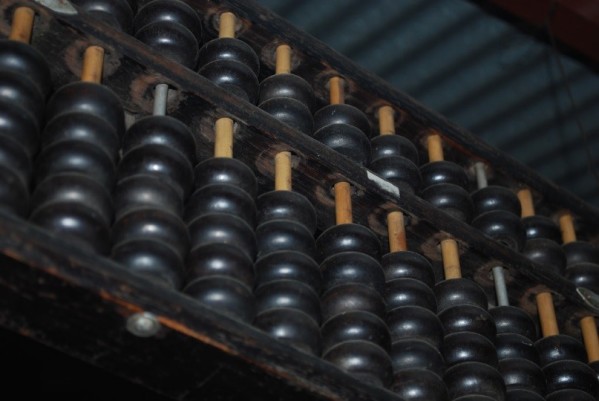
First-generation monitoring tools simply took events and log entries and fed them to hapless ops personnel as alerts. The result: alert storms consisting of far too much information to be usable, where important information became lost in a sea of useless data.
The expanding definition of application in today’s digital world is only adding to the noise. Instead of the monolithic applications of yesterday, today’s modern digital application is likely to run both on-premises and in the cloud, and consist of many separate elements: containers running numerous microservices, third-party plugins, and more.
This expanding context for the application is giving rise to a new market category: Digital Performance Management (DPM). DPM recognizes that in order to meet the needs and expectations of end-users, every element in such complex, interconnected applications must perform properly, every time, at velocity.
Machine Learning to the Rescue
How, then, to identify the critical nuggets of valuable information in the sea of useless data? To the rescue: machine learning technologies that make the noise filters more context sensitive and ideally, able to learn over time.
But even these next-generation monitoring technologies fall short, as they all operate after the fact. One problem might cause another and another in turn, and eventually, an admin gets an alert. Now they have to figure out the root cause of the issue.
The next generation of monitoring technology, therefore, focuses on root cause analysis. String together a sequence of related significant alerts over time and trace back to the earliest one. Determine both whether that earliest event is in fact the cause of the visible issues, and in addition, uncover the solution to the underlying problems.
Simple algorithmic machine learning, unfortunately, is generally not up to the task of identifying root causes in real-world, complex enterprise environments. Once again, vendors must step up their game, building out more sophisticated artificial intelligence (AI) capabilities that leverage advanced approaches like deep learning and big data analysis techniques to reveal that most precious of data points, the true root cause of complex problem scenarios.
Beyond Simple Machine Learning
With such “AI Ops” tools in their tool belts, ops personnel can now jump on problems quickly, as vendors rush to achieve the full vision of automated DPM. The missing piece: predictive analytics.
Predictive analytics raises the bar on AI-driven monitoring once more. It’s no longer good enough to nip problems in the bud. Now we want to predict problems before they occur so that we can prevent them from impacting operations in the first place.
Simple examples of such predictive capabilities are easy to envision. For example, if the memory utilization on a particular server continues to go up, eventually crossing a warning threshold, we can safely assume that the server will run out of memory in short order.
With complex digital applications, in contrast, such predictive ability pushes the limits of our AI and big data capabilities. Innovation, however, continues to proceed rapidly, and predictive analysis is rapidly improving as we speak.
The Intellyx Take
I mentioned the term AI Ops, but I’m reluctant to use it, as Gartner has muddied the waters about the meaning of this term. Even though AI stands for artificial intelligence, Gartner uses AIOps (without a space) to stand for Algorithmic IT Operations.
Algorithms, however, are nothing new, and nothing special. Gartner correctly defines an algorithm as “a set of rules that precisely defines a sequence of operations” – which would include everything from simple arithmetic to your grandmother’s muffin recipe.
Simple machine learning is in fact algorithmic – but as AI advances, the rules that define the sequence of operations become increasingly imprecise. Instead, they evolve on their own and become context-dependent, leading to the confusing result that AIOps as Gartner defines it falls short of AI-driven ops, as AI evolves to become post-algorithmic.
Vague analyst buzzwords aside, there’s no question that rapid innovation the field of AI is driving corresponding innovation in DPM. And regardless of whether you buy Gartner’s opinions about algorithms, we’re well on the road to automated, AI-driven operations.
Copyright © Intellyx LLC. Dynatrace is an Intellyx client. At the time of writing, none of the other organizations mentioned in this paper are Intellyx clients. Intellyx retains full editorial control over the content of this paper. Image credit: Scott.
Looking for answers?
Start a new discussion or ask for help in our Q&A forum.
Go to forum