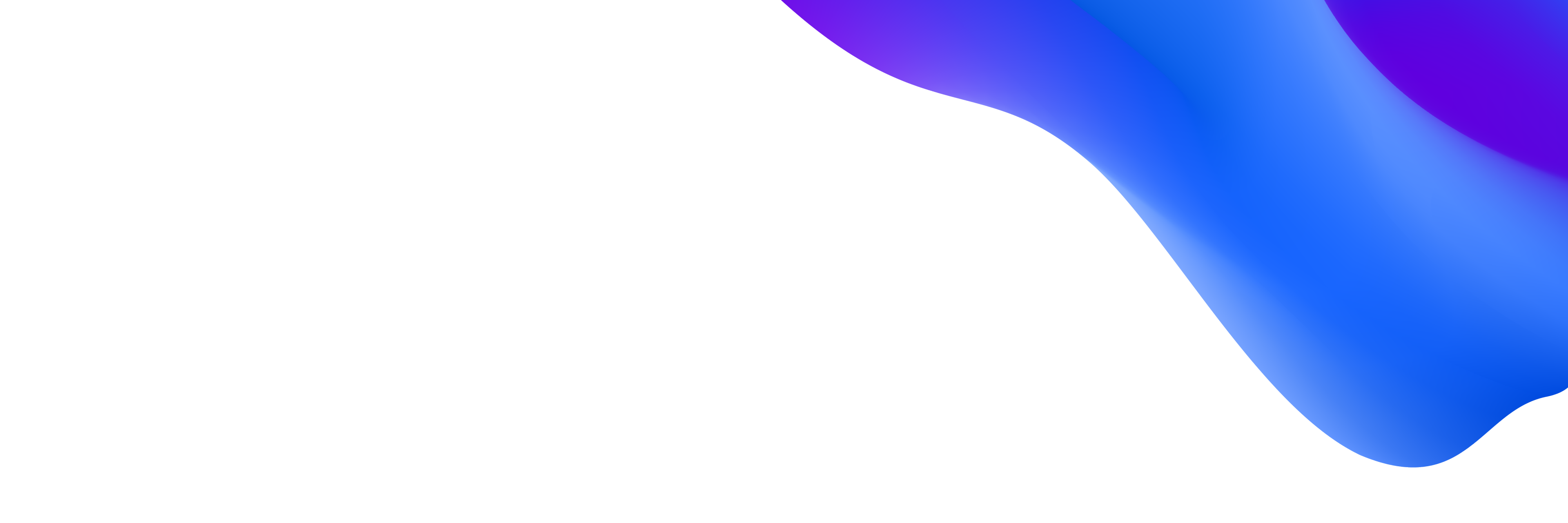
Data observability is a discipline that aims to address the needs of organizations to ensure data availability, reliability, and quality throughout the data lifecycle—from ingestion to analytics and automation.
Ensuring data trustworthiness and security can pose significant hurdles for organizations that rely on data to inform business and product strategies, optimize and automate processes, and drive continuous improvements.
Data observability can help identify, alert, troubleshoot, and resolve data issues in real time. As a result, organizations can be confident that the data they use for decision making, process automation, and operational strategies is high quality, timely, and accurate.

The following are key criteria to enable effective data observability:
Freshness. Ensures the data used for analytics and automation is up to date by alerting teams to any issues with data freshness, based on the behaviors detected with the Dynatrace platform’s Davis® causal and predictive AI capabilities.
Volume. Monitors for unexpected increases or decreases in the volume of data ingested into the analytics platform—for example, the number of reported customers using a particular service—which can be indicators of undetected issues.
Distribution. Monitors for patterns, outliers, and anomalies in data, looking for deviations from the expected distribution, which can signal issues in data collection or processing.
Schema. Tracks data structure and alerts on unexpected changes, such as new or deleted fields, to prevent unexpected outcomes like broken reports and dashboards, or projects that require extra analytics.
Lineage. Delivers precise root-cause detail into the origins of data and the services it will affect downstream, helping teams proactively identify and resolve data issues before they affect users or customers.