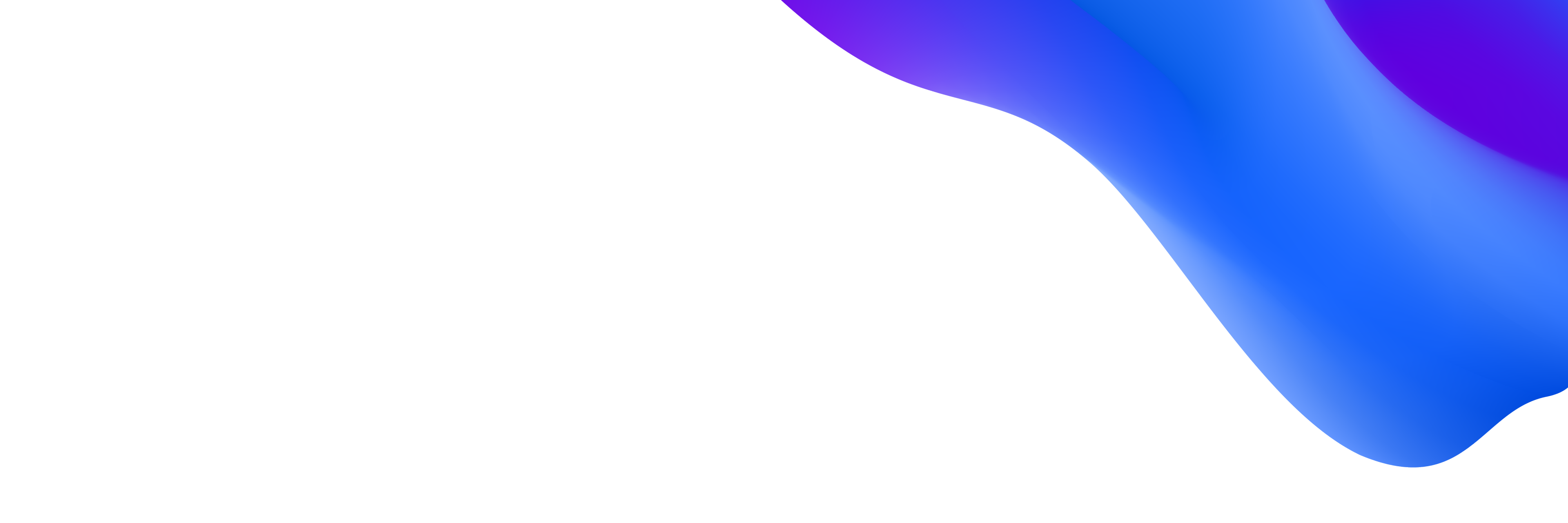
AI observability is the practice of applying monitoring and continuous analysis techniques to gain real-time insights into AI systems' behavior and performance. It's a critical aspect of building and maintaining reliable, transparent, and accountable AI systems that give developers, data scientists, and operations teams the information they need to ensure AI models are performant, cost-efficient, and fair.
Key components of AI observability
AI systems continue to grow in complexity, which means today’s organizations need a holistic approach to the observability of AI-powered cloud applications to ensure everything is working as it should.
Modern observability solutions offer a view into the application and infrastructure layers of a system. The application layer allows teams to monitor an application’s availability, latency, and reliability, while the infrastructure layer provides a view into infrastructure data, such as utilization, saturation, and errors.
AI observability goes a step further, painting a complete picture of the AI stack, which includes the following layers:
Orchestration layer. With AI observability, teams can get detailed workflow analysis, as well as insight into resource allocation and end-to-end execution from prompt to response.
Semantic layer. AI observability eliminates some of the challenges introduced by retrieval-augmented generation (RAG), enabling teams to monitor semantic caches and vector databases. Teams can better understand the effectiveness of RAG architecture from both retrieval and generation.
Model layer. Model observability enables teams to monitor resource consumption and operation costs. This gives organizations visibility into service-level performance metrics such as latency, availability, and errors.
Reap the rewards of AI observability
AI observability provides critical insights into model performance, helps to ensure compliance with service-level objectives, and facilitates AI applications' ongoing improvement. An AI observability solution should estimate and optimize costs, improve service quality, and ensure service reliability.
Learn more about how Dynatrace combines predictive, causal, and generative AI for observability, security, and business use cases.